Mar. 15, 2019 Research Highlight Physics / Astronomy
A neural network model partly explains how we store and recollect memories
A neural network model could provide a better understanding of how human memory works
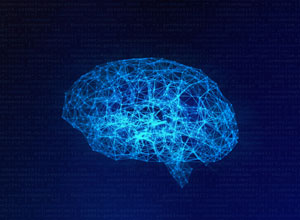
A mathematical model that provides insights into the mechanisms underlying the storage and retrieval of memories through oscillatory information coding has been developed by a pair of RIKEN researchers, opening a route to gaining a better understanding of how our brains work1.
Neurons are brain cells that process information. While the membranes that surround most cells are electrical insulators, neuron membranes contain channels that can transport electrically charged ions. These channels can be switched between an open and a closed state by altering the voltage across the membrane. The neurons form an interconnected network, passing these signals from one cell to the next via connections known as synapses. This concept is simple enough, but how human thought and memory emerges from this remains unclear.
The hippocampus is the brain region associated with the transfer of information from short-term to long-term memory and its subsequent retrieval. Recent experimental evidence has suggested that memories are consolidated in the hippocampus by the sequential reactivation of the hippocampal cells. This process, which is known as replay, is believed to be triggered by oscillations in the brain called sharp wave–ripples.
To gain further insight into this process, Chi Chung Alan Fung and Tomoki Fukai from the RIKEN Center for Brain Science have described it mathematically using a model known as a continuous attractor neural network. Attractor networks describe how interconnected neurons settle back into a stable pattern over time following an external stimulus. They have previously been applied to model the continuous information processing that occurs in an awake brain. However, a recent experimental study has indicated that in memory expression based on sharp wave–ripples, which occurs while we are resting or sleeping, information processing is not continuous, but occurs in discrete bursts.
Fung and Fukai used a perturbative approach to the neural field dynamics of the continuous attractor neural network to show that increasing the speed of an external stimulus causes the model to transition from a continuous state to discontinuous states. This transition gave a discrete-attractor-like behavior similar to that observed in the experiment.
“We have showed that a neuronal network that can represent continuous information can behave like a system with discrete memory under some conditions,” explains Fung. “It came as a surprise to us that a rather simple neural model with uniform settings can behave like a neural network with discrete attractors.”
The team now intends to use the model to study how discrete replay sequences of self-locations can benefit the information transfer across different brain regions during memory consolidation.
Related contents
References
- 1. Fung, C.C.A. & Fukai, T. Discrete-attractor-like tracking in continuous attractor neural networks. Physical Review Letters 122, 018102 (2019). doi: 10.1103/PhysRevLett.122.018102