Aug. 30, 2023 Research Highlight Physics / Astronomy
Gaining time without sacrificing accuracy when predicting typhoon-induced storm surges
Deep learning allows storm surges produced by typhoons to be quickly and accurately forecasted
A deep learning method developed by RIKEN researchers can accurately predict the destructive power of typhoon-induced storm surges on coastal regions in a fraction of the time of conventional models1. It promises to buy precious time for coastal residents without compromising accuracy.
The powerful winds and torrential rain generated by typhoons can wreak destruction and loss of life in inland regions. But coastal regions bear the full brunt of their destructive power through a phenomenon known as storm surges. The combination of strong winds and low pressure causes the sea level to rise well above its normal level, resulting in inundation of low-lying areas.
“When the bulge in the water surface reaches the coastline, it can cause flooding similar to that of tsunamis but spread out over two or three days,” explains Iyan Mulia of the RIKEN Prediction Science Laboratory.
Currently, two kinds of computer models are used to forecast wind and pressure fields for simulating storm surges. Parametric models use statistical calculations determined from past observations. They are quick to perform but can be unreliable under certain conditions. In contrast, numerical weather prediction (NWP) models, which account for the physics involved, are more accurate but take much more time and computing power to perform.
“Parametric models are computationally more efficient but less accurate than NWP models,” says Mulia. “Our goal is to develop a model that is as efficient as parametric models but as accurate as NWP models.”
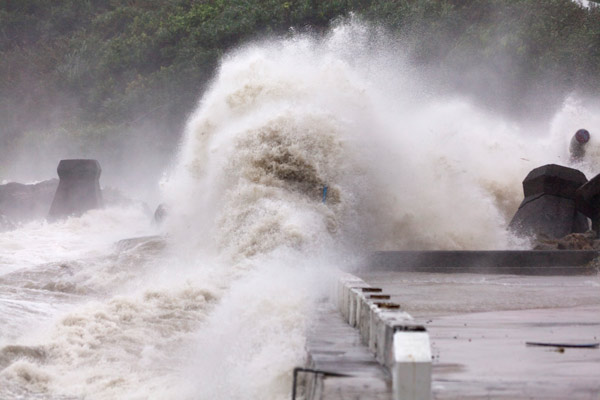
Figure 1: Storm surges that are produced by typhoons can cause great devastation and loss of life in coastal areas. © 2023 JIM EDDS/SCIENCE PHOTO LIBRARY
Now, Mulia and his co-workers have used deep learning to combine the advantages of both models. “Our deep learning model can produce comparable accuracy to NWP models with significantly less computational effort,” says Mulia.
Their model uses deep learning to convert the results of a parametric model into realistic wind and pressure fields within a few seconds on a normal desktop computer. These are then used to calculate the effect on the sea. The team employed this approach because it is much more demanding to calculate atmospheric conditions than water ones.
Even Mulia was surprised at how well the deep learning model performed. “We didn’t expect that the deep learning model could achieve such high accuracy given how complex typhoon structures are,” he says. “For instance, it clearly captures the topographic effect on the wind, which is difficult to achieve using standard parametric models. We’re very happy with the result.”
More testing using larger data sets is needed before the method can be deployed in operational forecasting systems, Mulia notes.
The team is now applying the same approach to other fields such as environmental science and engineering.
Related contents
- Deep learning can predict tsunami impacts in less than a second
- New simulation method allows for more accurate assessment of regional climate
Rate this article
Reference
- 1. Mulia, I. E., Ueda, N., Miyoshi, T., Iwamoto, T. & Heidarzadeh, M. A novel deep learning approach for typhoon-induced storm surge modeling through efficient emulation of wind and pressure fields. Scientific Reports 13, 7918 (2023). doi: 10.1038/s41598-023-35093-9