Dec. 26, 2023 Perspectives Computing / Math
Turning the tables on weather forecasting
With unprecedented supercomputing power, new data and AI, we are on the threshold of highly accurate weather prediction—and maybe even control, explains Takemasa Miyoshi.
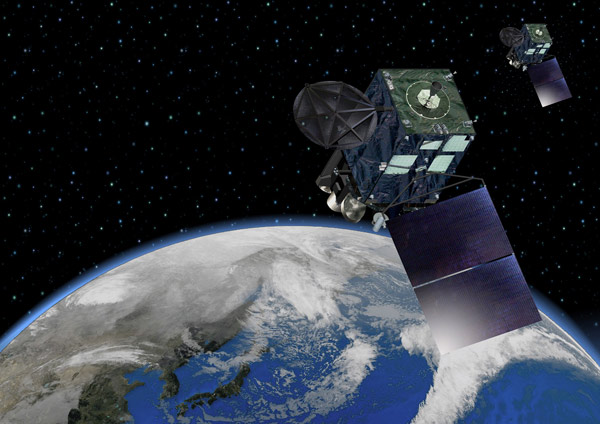
RIKEN researchers have been using infrared images from Japan's Himawari-8/9 geostationary satellites (pictured) to develop a system to provide flood and river discharge warnings. By assimilating the satellite images every 10 minutes, the team have shown they can monitor rapidly changing severe weather. Japan Meteorological Agency, modified and licensed under CC BY 4.0
The basic equations used in meteorology have remained fundamentally unchanged since they were developed by Norwegian physicist Vilhelm Bjerknes in the early 1900s, based on calculations of atmospheric dynamics. Progress in weather prediction since then has principally been in the enormous expansion of weather monitoring resources around the world and in the supercomputers we use to solve the equations that use this influx of data.
We also now have the ability to combine newer observation technologies, including ground-based radar, satellite imagery and meteorological readings, to give an incredibly accurate snapshot of current conditions across most parts of the world. So the true challenge of weather prediction today is assimilating all of that data and running sufficient numbers of simulations quickly enough to enable timely prediction updates.
At the RIKEN Center for Computational Science, we are fortunate to have access to the Fugaku supercomputer, one of the most powerful computing facilities in the world. Using even a small fraction of Fugaku’s power has allowed us to demonstrate what is possible at the cutting edge of weather prediction science.
An Olympic demonstration
For the 2020 summer olympics, and paralympics, held in Tokyo in 2021 due to the COVID-19 pandemic, our group collaborated with researchers from other Tokyo institutions to develop a software system to move data from an advanced radar system direct to Fugaku, in order to deliver high-resolution coverage of the region within three seconds.
Working with computer scientists, we developed software that exploited Fugaku’s full power by tailoring the data interconnections among its processing nodes to optimize computational speed.
Using just 7% of the available processing nodes, we were able to assimilate data from the radar and complete 1,000 parallel ‘ensemble’ simulations in just 15 seconds, allowing for a weather prediction update every 30 seconds.
The ensemble simulation approach is how we account for uncertainty in our predictions. We take the current set of observations and run multiple simulations with slight variations to the initial conditions and model a number of assumptions to generate multiple scenarios. The challenge for this olympic project was creating a computational environment that allowed us to complete the 1,000 simulations in the ensemble in an extremely short time period.
What was really groundbreaking about this prediction system was modeling the development of small individual ‘convective clouds’ for the first time. These are billowing, tall, rain-bearing clouds that form quickly when warm humid air rises through cooler surrounding air, typically in humid conditions.
Usually, the resolution of weather models is in the order of kilometers. But the resolution needed to model convective clouds is smaller than this. Weather models are also often only updated hourly, while these clouds can form, dissipate and evolve significantly in just minutes.
Our system, which ran for the duration of the olympics and paralympics, generated a 3D model of convective clouds every 30 seconds, and predicted the evolution and rain for the following half an hour. The model was accessible on a smartphone app developed with a partner for this purpose.
This demonstration showed the power of what is now possible using the latest technology, and it has been recognized by our nomination as a finalist for the 2023 Gordon Bell Prize for Climate Modelling. This prize is awarded annually by the world’s largest computing association, the Association for Computing Machinery, to recognize outstanding achievement in high-performance computing1.
We have also been working on early warning of flood risk, flood development and river discharge with the integration of data from Japan's Himawari-8/9 geostationary satellites. These satellites capture regular infrared images covering the entirety of Japan. By assimilating this data every 10 minutes, we have shown that it is possible to monitor the flood risk associated with rapidly changing severe weather2 .
Not only can this data provide warning of floods, it can improve the analysis of moisture transport and modeling of strong precipitation bands in the models we use for prediction.
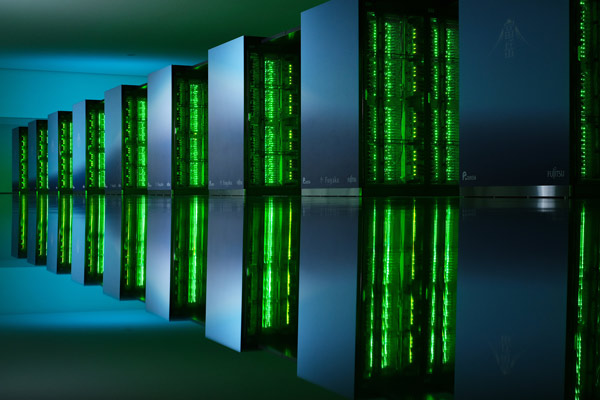
In 2021, the Fugaku supercomputer (pictured) was used to complete 1,000 parallel high-resolution weather simulations in 15 seconds, allowing a Tokyo weather update every 30 seconds. © 2023 RIKEN
AI efficiency
One of the problems with our current ensemble simulation approach for weather prediction is that it is computationally intensive and requires nationally-supported infrastructure to be available to the public. This type of investment is not possible in many countries.
Even in Japan, the current operational weather prediction run by the Japan Meteorological Agency is updated once every hour at a two-kilometer resolution and uses a relatively limited dataset. Upgrading this operational system to something like our olympic demonstration would require further investment in infrastructure and a permanent tenancy on Fugaku.
We are beginning to turn our attention to AI as a potentially more efficient and accessible platform for weather prediction. Could an AI model running on a single desktop graphics processing unit (GPU) predict the weather with comparable accuracy to what we currently achieve using supercomputers?
This is a very important question that is being explored. But we still need data—AI is only as good as the data it is trained on—but assuming we can train an AI model on a sufficiently large and complete dataset, we believe this revolution could be possible and would put sophisticated prediction in the hands of the people.
Harnessing uncertainty for control
The key to prediction is uncertainty: those of us pushing the limits of weather prediction are consumed by it. In our ensemble simulations, each simulation is equally probable, so the more of these simulations we run for each point in time, the better out modeling is at representing uncertainty.
However, regardless of the number of ensemble simulations, all predictions become increasingly uncertain as they move further into the future, through an accumulation of more possible consequences.
What this ensemble approach also shows us is that the tiniest fluctuation in any of the starting conditions or model assumptions can result in very different weather evolutions over time, also known as the ‘butterfly effect’.
This is a bane for forecasting, but it also raises an intriguing and potentially profound question: If the tiniest change can affect weather development so significantly in a simulation, could we not make that small modification in the real world to change the evolution of weather itself?
With our new ability to run thousands of ensemble simulations using high-resolution data, it is now feasible to accurately model the likely consequences of such a deliberate change to the weather.
My team has shown that it is technically feasible to run very large ensembles quickly to ‘experiment’ with the weather virtually, such as the consequences of small wind changes on the severity of typhoons or heavy rains. We think these experiments could one day lead to targeted weather modifications that could, for example, prevent the development of a predicted extreme event3. AI could help pinpoint the most efficient modifications.
This of course is not something that could, or should, be undertaken lightly. Great care needs to be taken that our control efforts don’t have unintended consequences. But perhaps this is a way to turn the tables, to mitigate some of the weather impacts that we have caused as a result of climate change.
Our simulation sandbox is a great place to perform such experiments safely.
Rate this article
Related links
- 1. Eyes beyond the prize (accessed 26 September, 2023).
- 2. Honda, T. Kotsuki, S., Lien, G.-Y., Maejima, Y., Okamoto, K. & Miyoshi, T. Assimilation of Himawari-8 All-Sky Radiances Every 10 Minutes: Impact on Precipitation and Flood Risk Prediction . Journal of Geophysical Research 123, 965-976 (2018). doi: 10.1002/2017JD027096
- 3. Miyoshi, T. & Sun, Q. Control Simulation Experiment with the Lorenz's Butterfly Attractor. Nonlinear Processes in Geophysics 29, 133-139 (2022). doi: 10.5194/npg-29-133-2022
About the researcher
Takemasa Miyoshi, Team leader, Data Assimilation Research Team, RIKEN Center for Computational Science
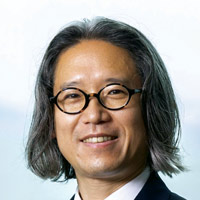
Takemasa Miyoshi started his professional career as a civil servant at the Japan Meteorological Agency (JMA) in 2000. In 2005, he gained his PhD at the University of Maryland (UMD) in the United States. He then became a tenure-track assistant professor at UMD in 2011. Since 2012, Miyoshi has led the Data Assimilation Research Team at the RIKEN Center for Computational Science (R-CCS). There he is working towards advancing science on data assimilation. Miyoshi has been recognized by several prestigious awards. These include the Meteorological Society of Japan Award (2016), the Yomiuri Gold Medal Prize (2018), a commendation by the Prime Minister for disaster prevention (2020), and an award for science and technology by the Minister of Education, Culture, Sports, Science and Technology (2022).