Mar. 5, 2024 Research Highlight Physics / Astronomy
AI tool reveals the orientation of nuclei in heavy-ion collisions
The orientation of deformed atomic nuclei in particle collisions can be determined using a newly developed neural network
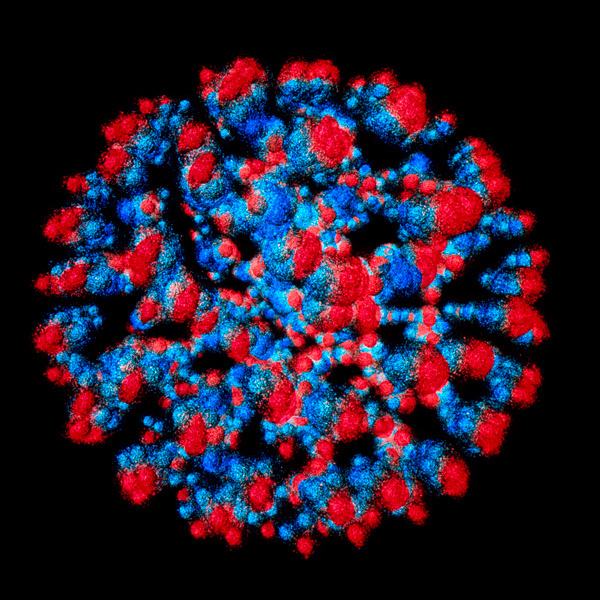
Figure 1: Computer visualization of an uranium nucleus showing its quark structure. Uranium nuclei are usually spherical, but they can become flattened when accelerated to high velocities in colliders. A neural network developed by RIKEN researchers can analyze the orientation of nuclei in collisions. © ARSCIMED/SCIENCE PHOTO LIBRARY
Horizontal pancakes or upright cigars? A new artificial intelligence (AI) tool developed by RIKEN physicists will help to determine which of these two orientations best describes the nuclei inside heavy atoms that are smashed together in particle accelerators1. This promises to provide a new window into the properties of nuclei.
Particle physicists can recreate conditions similar to those in the early Universe by smashing together massive atoms, such as uranium, at high energies in heavy-ion colliders. By using computational tools to analyze the collision debris, they can infer the properties of the original nuclei, including their orientations.
A few decades ago, the orientations of nuclei didn’t factor into the analysis. “Before 2000, nuclear physicists assumed that the nuclei in the collisions were spherical,” says Zu-Xing Yang, a physicist at the RIKEN Nishina Center for Accelerator-Based Science.
But then physicists realized that the atomic nuclei are actually squashed or stretched along one direction or another. This deformation is thought to be caused by the motion and interactions of a subset of protons and neutrons inside each nucleus.
That means that incoming nuclei can be oriented in different ways relative to each other when they collide. Nuclei may be oriented as flat pancakes or as upright cigars, for instance.
The question was whether the orientation could be determined for a single collision event, says Yang.
To help uncover the answer, Yang and three colleagues have created an AI tool—a neural network similar to those used in facial-recognition software.
“Neural networks should be powerful for addressing such complex problems,” says Yang, noting that they have been successfully deployed to analyze other collision parameters in the past.
The team trained their neural network using simulations of collisions between uranium ions. Once trained, the neural network was able to correctly map from the final states after collision to the initial orientations of the original incoming nuclei, in more than 70% of simulated cases.
This accuracy is high enough for the tool to be employed to learn more about the specific orientations of nuclei. “This holds significance for studying nuclear properties,” says Yang.
The next step is to use the AI tool to analyze real experimental data. Some data is already available since such uranium–uranium smash-ups were conducted at the Relativistic Heavy Ion Collider at the Brookhaven National Laboratory in New York in 2015. Similar experiments are also planned at RIKEN and in China.
“We’re looking forward to physicists applying our tool to experimental data,” says Yang.
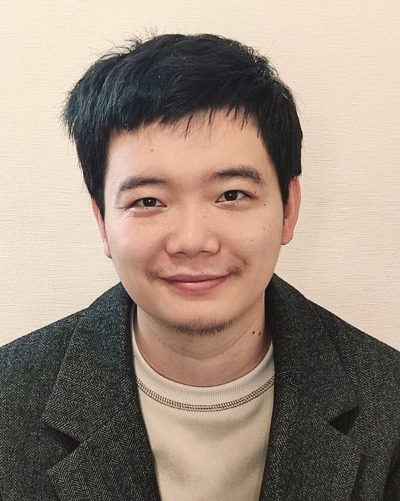
Zu-Xing Yang and colleagues have developed a neural network to analyze the orientation of deformed atomic nuclei in heavy-ion collisions. © 2024 RIKEN
Related content
- Measuring the lifetimes of strange nuclei
- Probing the properties of vacuum in nuclei
- Stuffing sodium nuclei with neutrons
Rate this article
Reference
- 1. Yang, Z.-X., Fan, X.-H., Li, Z.-P. & Nishimura, S. A neural network approach for orienting heavy-ion collision events. Physics Letters B 848, 138359 (2024). doi: 10.1016/j.physletb.2023.138359