RIKEN Center for Advanced Intelligence Project Approximate Bayesian Inference Team
Team Leader: Mohammad Emtiyaz Khan (Ph.D.)
Research Summary
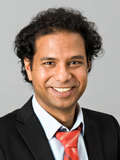
Humans, animals, and other living beings have a natural ability to autonomously learn throughout their lives and quickly adapt to their surroundings, but AI lack such abilities. Our goal is to bridge such gaps between the learning of living-beings and AI. We are machine learning researchers with an expertise in areas such as approximate inference, Bayesian statistics, continuous optimization, information geometry etc. We work on a variety of learning problems, especially those involving supervised, continual, active, federated, online, and reinforcement learning. Currently, we are developing algorithms which enable computers to autonomously learn to perceive, act, and reason throughout their lives.
Main Research Fields
- Computer Science
Research Subjects
- Machine Learning
- Statistics
- Artificial Intelligence
Selected Publications
- 1.
Mollenhoff T. and Khan, M. E.
"SAM as an Optimal Relaxation of Bayes"
International Conference on Learning Representation, to appear (2023). - 2.
Khan, M. E. and Swaroop, S.
"Knowledge-adaptation priors"
Advances in Neural Information Processing Systems 34, pp. 19757-19770, (2021). - 3.
Pan, P., Swaroop, S., Immer, A., Eschenhagen, R., Turner, R. and Khan, M. E.
"Continual deep learning by functional regularization of the memorable past"
Advances in Neural Information Processing Systems, to appear (2020). - 4.
Tomasev, N. et al.
"AI For social good: unlocking the opportunity for positive impact"
Nature communications, 11, 2468 (2020). - 5.
Meng, X., Bachmann, R. and Khan, M. E.
"Training binary neural networks using the Bayesian Learning rule"
Proceedings of the 37th International Conference on Machine Learning, PMLR 119, pp. 6852-6861, (2020). - 6.
Osawa, K., Swaroop, S., Khan, M. E., Jain, A., Eschenhagen, R., Turner, R. E. and Yokota, R.
"Practical Deep Learning with Bayesian Principles"
Advances in Neural Information Processing Systems 32, pp. 4287?4299 (2019). - 7.
Khan, M. E., Immer, A., Abedi, E. and Korzepa, M.
"Approximate Inference Turns Deep Networks into Gaussian Processes"
Advances in Neural Information Processing Systems 32, pp. 3094?3104 (2019). - 8.
Cherief-Abdellatif, B.-E., Alquier, P. and Khan, M. E.
"A Generalization Bound for Online Variational Inference"
Proceedings of The 11th Asian Conference on Machine Learning, PMLR 101, pp. 662-677 (2019). - 9.
Lin, W., Khan, M. E. and Schmidt, M.
"Fast and Simple Natural-Gradient Variational Inference with Mixture of Exponential-family Approximations"
Proceedings of the 36th International Conference on Machine Learning, PMLR 97, pp. 3992-4002 (2019). - 10.
Khan, M. E. and Lin, W.
"Conjugate-Computation Variational Inference : Converting Variational Inference in Non-Conjugate Models to Inferences in Conjugate Models"
Proceedings of the 20th International Conference on Artificial Intelligence and Statistics, PMLR 54, pp. 878-887 (2017).
Related Links
Lab Members
Principal investigator
- Mohammad Emtiyaz Khan
- Team Leader
Core members
- Thomas Moellenhoff
- Research Scientist
- Keigo Nishida
- Special Postdoctoral Researcher
- Hugo Monzon
- Postdoctoral Researcher
- Zhedong Liu
- Postdoctoral Researcher
- Christopher Anders
- Postdoctoral Researcher
- Yohan Jung
- Postdoctoral Researcher
- Pierre Alquier
- Visiting Scientist
- Joseph Austerweil
- Visiting Scientist
- Rio Yokota
- Visiting Scientist
- Dharmesh Vijay Tailor
- Student Trainee
- Anita Yang
- Research Part-time Worker I
- Clement Bazan
- Research Part-time Worker II
Contact Information
Nihonbashi 1-chome Mitsui Building, 15th floor,
1-4-1 Nihonbashi,
Chuo-ku, Tokyo
103-0027, Japan
Email: emtiyaz.khan [at] riken.jp