RIKEN Center for Advanced Intelligence Project Continuous Optimization Team
Team Director: Akiko Takeda (D.Sc.)
Research Summary
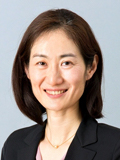
Our team focuses on mathematical optimization. An optimization problem is the problem of finding the best solution from all feasible solutions. By formulating various real-world problems as optimization problems and solving them by efficient algorithms, we can find their reasonable solutions. Optimization algorithms are applicable not only to machine learning problems but also to various applications, e.g., of production research and industrial engineering. However some problems such as nonconvex optimization and uncertain optimization problems are difficult to solve. Currently, we are working on efficient algorithms for such difficult optimization problems.
Research Subjects:
- Mathematical Optimization
- Nonconvex Optimization
- Uncertainty
Main Research Fields
- Informatics
Related Research Fields
- Interdisciplinary Science & Engineering
- Mathematical informatics
Keywords
- Mathematical Optimization
- Operations Research
Selected Publications
- 1.
Naoki Marumo, Takayuki Okuno, Akiko Takeda.:
"Accelerated-gradient-based generalized Levenberg--Marquardt method with oracle complexity bound and local quadratic convergence"
Mathematical Programming, 2024 [Advance online publication]. - 2.
Jan Harold Alcantara, Chieu Thanh Nguyen, Takayuki Okuno, Akiko Takeda and Jein-Shan Chen.:
"Unified Smoothing Approach for Best Hyperparameter Selection Problem Using a Bilevel Optimization Strategy"
Mathematical Programming, 2024 [Advance online publication]. - 3.
Naoki Marumo, Akiko Takeda.:
"Parameter-free accelerated gradient descent for nonconvex minimization"
SIAM Journal on Optimization, 34(2), pp. 2093--2120 (2024). - 4.
Tianxiang Liu, Ting Kei Pong, Akiko Takeda.:
"Doubly majorized algorithm for sparsity-inducing optimization problems with regularizer-compatible constraints"
Computational Optimization and Applications, 86, pp. 521-–553 (2023). - 5.
Pierre-Louis Poirion, Bruno F. Lourenco, Akiko Takeda.:
"Random projection of Linear and Semidefinite problem with linear inequalities"
Linear Algebra and Its Applications, 664, pp. 24--60 (2023). - 6.
Hidenori Iwakiri, Yuhang Wang, Shinji Ito, Akiko Takeda.:
"Single Loop Gaussian Homotopy Method for Non-convex Optimization"
Advances in Neural Information Processing Systems 35, pp. 7065--7076 (2022). - 7.
Michael Metel, Akiko Takeda.:
"Perturbed Iterate SGD for Lipschitz Continuous Loss Functions"
Journal of Optimization Theory and Applications, 195, pp.504--547 (2022). - 8.
Mitsuaki Obara, Takayuki Okuno, Akiko Takeda.:
"Sequential Quadratic Optimization for Nonlinear Optimization Problems on Riemannian Manifolds"
SIAM Journal on Optimization, 32(2), pp.822-853 (2022). - 9.
Terunari Fuji, Pierre-Louis Poirion, and Akiko Takeda.:
"Convexification with bounded gap for randomly projected quadratic optimization"
SIAM Journal on Optimization, 32(2), pp.874-899 (2022). - 10.
Ryo Sato, Mirai Tanaka, Akiko Takeda.:
"A Gradient Method for Multilevel Optimization"
Advances in Neural Information Processing Systems 34, pp. 7522--7533 (2021).
Related Links
Lab Members
Principal investigator
- Akiko Takeda
- Team Director
Core members
- Pierre-Louis Poirion
- Research Scientist
- Jan Harold Alcantara
- Postdoctoral Researcher
- Trung Hieu Vu
- Postdoctoral Researcher
- Mitsuhiro Nishijima
- Postdoctoral Researcher
- Benjamin Poignard
- Visiting Scientist
- Andi Han
- Visiting Scientist
- Mitsuaki Obara
- Research Part-time Worker I
Contact Information
Department of Creative Informatics,
Graduate School of Information Science and Technology,
The University of Tokyo
7-3-1 Hongo, Bunkyo-ku, Tokyo
113-8656, Japan
Email: akiko.takeda@riken.jp