RIKEN Center for Advanced Intelligence Project Deep Learning Theory Team
Team Director: Taiji Suzuki (Ph.D.)
Research Summary
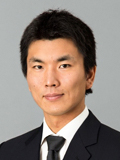
Our team, deep learning theory team, is studying various kinds of learning systems including deep learning from theoretical viewpoints. We enrich our understandings of complex learning systems, and leverage the insights to construct new machine learning techniques and apply them. Especially, machine learning should deal with high dimensional and complicated data, and thus we are studying deep learning and structured sparse learning as methods to deal with such complicated data. Moreover, we are also developing efficient optimization algorithms for large and complicated machine learning problems based on such techniques as stochastic optimization.
Research Subjects:
- Statistical learning theory of wide range of learning systems including deep learning
- Efficient optimization algorithm for large dataset
- High dimensional statistics
Main Research Fields
- Informatics
Related Research Fields
- Mathematical & Physical Sciences
- Principles of Informatics/Mathematical informatics
- Principles of Informatics/Statistical science
Keywords
- Deep learning
- Statistical learning theory
- Machine learning
- Stochastic optimization
- Mathematical statistics
Selected Publications
- 1.
Juno Kim, Taiji Suzuki.:
"Transformers Learn Nonlinear Features In Context: Nonconvex Mean-field Dynamics on the Attention Landscape. Forty-first International Conference on Machine Learning (ICML2024),"
Proceedings of Machine Learning Research, 235:24527--24561, (2024) - 2.
Kazusato Oko, Shunta Akiyama, Denny Wu, Tomoya Murata, Taiji Suzuki.:
"SILVER: Single-loop variance reduction and application to federated learning. Forty-first International Conference on Machine Learning" (ICML2024)
Proceedings of Machine Learning Research, 235:38683--38739, (2024) - 3.
Taiji Suzuki, Denny Wu, Atsushi Nitanda.:
"Convergence of mean-fieldLangevin dynamics: Time and space discretization, stochastic gradient,and variance reduction."
Thirty-seventh Conference on NeuralInformation Processing Systems (NeurIPS2023), pp. 15545--15577,(2023) - 4.
Taiji Suzuki, Denny Wu, Kazusato Oko, Atsushi Nitanda.:
"Feature learning via mean-field Langevin dynamics: classifying sparse parities and beyond."
Thirty-seventh Conference on Neural Information Processing Systems (NeurIPS2023), pp. 34536--34556,(2023) - 5.
Kazusato Oko, Shunta Akiyama, Taiji Suzuki.:
"Diffusion Models are Minimax Optimal Distribution Estimators. Proceedings of the 40th International Conference on Machine Learning (ICML2023)"
Proceedings of Machine Learning Research, 202:26517--26582,(2023) - 6.
Shokichi Takakura, Taiji Suzuki.:
"Approximation and Estimation Ability of Transformers for Sequence-to-Sequence Functions with Infinite Dimensional Input. Proceedings of the 40th International Conference on Machine Learning (ICML2023)"
Proceedings of Machine Learning Research, 202:33416--33447, (2023) - 7.
Atsushi Nitanda, Denny Wu, Taiji Suzuki.:
"Convex Analysis of the Mean Field Langevin Dynamics. 25th International Conference on Artificial Intelligence and Statistics (AISTATS2022)"
Proceedings of Machine Learning Research, 151:9741--9757,(2022) - 8.
Atsushi Nitanda, and Taiji Suzuki.:
"Optimal Rates for Averaged Stochastic Gradient Descent under Neural Tangent Kernel Regime."
ICLR2021 (ICLR2021 outstanding paper award). - 9.
Taiji Suzuki, Hiroshi Abe, Tomoya Murata, Shingo Horiuchi, Kotaro Ito, Tokuma Wachi, So Hirai, Masatoshi Yukishima, Tomoaki Nishimura.:
"Spectral pruning: Compressing deep neural networks via spectral analysis and its generalization error"
The 29th International Joint Conference on Artificial Intelligence and the 17th Pacific Rim International Conference on Artificial Intelligence (IJCAI-PRICAI 2020) - 10.
Taiji Suzuki, Hiroshi Abe, Tomoaki Nishimura.:
"Compression based bound for non-compressed network: unified generalization error analysis of large compressible deep neural network"
The 8th International Conference on Learning Representations (ICLR 2020)
Related Links
Lab Members
Principal investigator
- Taiji Suzuki
- Team Director
Core members
- Sho Sonoda
- Senior Research Scientist
- Wei Huang
- Research Scientist
- Stefano Massaroli
- Postdoctoral Researcher
- Tomoya Wakayama
- Postdoctoral Researcher
- Takafumi Kanamori
- Visiting Scientist
- Takashi Takenouchi
- Visiting Scientist
- Hironori Fujisawa
- Visiting Scientist
- Shotaro Akaho
- Visiting Scientist
- Takayuki Kawashima
- Visiting Scientist
- Yuichiro Wada
- Visiting Scientist
- Noboru Murata
- Visiting Scientist
- Tomoya Murata
- Visiting Scientist
- Kazusato Oko
- Student Trainee
- Charles Freddy Pierre-Louis Vielzeuf
- Student Trainee
- Bingrui Li
- Student Trainee
- Dake Bu
- Intern
- Kosaku Takanashi
- Research Part-time Worker I
- Naoki Nishikawa
- Research Part-time Worker I
- Ryotaro Kawata
- Research Part-time Worker II
- Rei Higuchi
- Research Part-time Worker II
- Kento Kuwataka
- Research Part-time Worker II
Contact Information
Department of Mathematical Informatics, Graduate School of Information Science and Technology, The University of Tokyo
Hongo 7-3-1, Bunkyo-ku, Tokyo
113-8656, JAPAN
Email: taiji.suzuki@riken.jp