RIKEN Center for Advanced Intelligence Project Sequential Decision Making Team
Team Director: Shinji Ito (Ph.D.)
Research Summary
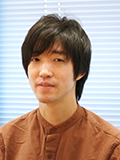
The Sequential Decision Making Team works to develop algorithms and theories for making rational decisions in a sequential manner in the face of forecast uncertainty and environmental fluctuations. In recent years, along with the evolution of information technology, there has been a demand for technology to make rational decisions based on the large amount of data being generated in real-time in today's world. To meet this challenge, we promote research related to online learning, bandit problems, and reinforcement learning, aiming to understand effective decision-making algorithms in a fluctuating environment and to construct and extend theoretical systems that support such algorithms.
Main Research Fields
- Informatics
Related Research Fields
- Engineering
- Mathematical & Physical Sciences
- Theory of informatics
- Mathematical informatics
- Intelligent informatics
Keywords
- Sequential decision-making
- Online learning
- Bandit problems
- Reinforcement learning
- Learning theory
Selected Publications
- 1.
S. Ito and K. Takemura:
"An Exploration-by-Optimization Approach to Best of Both Worlds in Linear Bandits"
Advances in Neural Information and Processing Systems 36 (NeurIPS), to appear (2023). - 2.
S. Ito, D. Hatano, H. Sumita, K. Takemura, T. Fukunaga, N. Kakimura, and K.-I. Kawarabayashi:
"Bandit Task Assignment with Unknown Processing Time“
Advances in Neural Information and Processing Systems 36 (NeurIPS), to appear (2023). - 3.
T. Tsuchiya, S. Ito, and J. Honda:
"Stability-penalty-adaptive follow-the-regularized-leader: Sparsity, game-dependency, and best-of-both-worlds“
Advances in Neural Information and Processing Systems 36 (NeurIPS), to appear (2023). - 4.
S. Ito and K. Takemura:
"Best-of-Three-Worlds Linear Bandit Algorithm with Variance-Adaptive Regret Bounds"
Proceedings of 36th Conference on Learning Theory (COLT), pp. 2653-2677 (2023). - 5.
T. Tsuchiya, S. Ito, and J. Honda:
"Further Adaptive Best-of-Both-Worlds Algorithm for Combinatorial Semi-Bandits"
Proceedings of The 26th International Conference on Artificial Intelligence and Statistics (AISTATS), pp. 8117-8144 (2023). - 6.
J. Honda, S. Ito, and T. Tsuchiya:
"Follow-the-Perturbed-Leader Achieves Best-of-Both-Worlds for Bandit Problems"
Proceedings of The 34th International Conference on Algorithmic Learning Theory (ALT), pp. 726-754 (2023). - 7.
T. Tsuchiya, S. Ito, and J. Honda:
"Best-of-Both-Worlds Algorithms for Partial Monitoring"
Proceedings of The 34th International Conference on Algorithmic Learning Theory (ALT), pp. 1484-1515 (2023). - 8.
S. Ito, T. Tsuchiya, and J. Honda:
"Nearly Optimal Best-of-Both-Worlds Algorithms for Online Learning with Feedback Graphs"
Advances in Neural Information and Processing Systems 35 (NeurIPS), pp. 28631-28643 (2022). - 9.
S. Ito:
"Revisiting Online Submodular Minimization: Gap-Dependent Regret Bounds, Best of Both Worlds and Adversarial Robustness"
Proceedings of the 39th International Conference on Machine Learning (ICML), pp. 9678-9694 (2022). - 10.
S. Ito, T. Tsuchiya, and J. Honda:
"Adversarially Robust Multi-Armed Bandit Algorithm with Variance-Dependent Regret Bounds"
Proceedings of 36th Conference on Learning Theory (COLT), pp. 1421-1422 (2022).
Related Links
Lab Members
Principal investigator
- Shinji Ito
- Team Director
Core members
- Junya Honda
- Visiting Scientist
- Taira Tsuchiya
- Visiting Scientist
- Junpei Komiyama
- Visiting Scientist
- Kazushi Tsutsui
- Visiting Scientist
- Tasuku Soma
- Visiting Scientist
- Taihei Oki
- Visiting Scientist
Contact Information
Nihonbashi 1-chome Mitsui Building, 15th floor,
1-4-1 Nihonbashi, Chuo-ku, Tokyo
103-0027, Japan
Email:shinji.ito.hh [at] riken.jp