RIKEN Center for Advanced Intelligence Project Functional Analytic Learning Team
Team Director: Minh Ha Quang (Ph.D.)
Research Summary
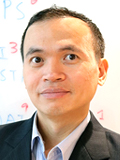
The Functional Analytic Learning Team focuses on theories and methods from functional analysis and related fields in machine learning, in particular methods based on reproducing kernel Hilbert spaces (RKHS), matrix and operator theory, Riemannian geometry, information geometry, and optimal transport. An important direction is the theoretical formulations and algorithms based on infinite-dimensional geometrical methods, especially in the RKHS setting. The targeted application domains include, but are not limited to, functional data analysis, computer vision, image and signal processing, brain imaging, and brain computer interfaces.
Research Subjects
- Vector-valued Reproducing Kernel Hilbert Spaces
- Geometrical methods in machine learning
Main Research Fields
- Informatics
Related Research Fields
- Mathematical & Physical Sciences
- Intelligent Informatics
- Mathematical Informatics
- Mathematical Analysis
Keywords
- Reproducing kernel Hilbert spaces
- Riemannian geometry
- Information geometry
- Optimal transport
- Gaussian processes
Selected Publications
- 1.
Ha Quang Minh.
"Fisher–Rao geometry of equivalent Gaussian measures on infinite-dimensional Hilbert spaces"
Information Geometry, June (2024) - 2.
Ha Quang Minh.
"Infinite-dimensional distances and divergences between positive definite operators, Gaussian measures, and Gaussian processes"
Information Geometry, May (2024) - 3.
Ha Quang Minh.
"Convergence and finite sample approximations of entropic regularized Wasserstein distances in Gaussian and RKHS settings"
Analysis and Applications, Vol. 21, No. 03, pp. 719-775 (2023) - 4.
Ha Quang Minh.
"Entropic regularization of Wasserstein distance between infinite-dimensional Gaussian measures and Gaussian processes"
Journal of Theoretical Probability, Volume 36, pages 201–296, (2023) - 5.
Ha Quang Minh.
"Alpha Procrustes metrics between positive definite operators: a unifying formulation for the Bures-Wasserstein and Log-Euclidean/Log-Hilbert-Schmidt metrics"
Linear Algebra and Its Applications, volume 636, pages 25-58, 2022 - 6.
Ha Quang Minh.
"Finite sample approximations of exact and entropic Wasserstein distances between covariance operators and Gaussian processes"
SIAM/ASA Journal on Uncertainty Quantification, volume 10, number 1, pages 96-124, 2022 - 7.
Anton Mallasto, ]Augusto Gerolin, H`a Quang Minh.
"Entropy-regularized 2-Wasserstein distance between Gaussian measures"
Information Geometry, volume 5, pages 289-323, 2022 - 8.
Ha Quang Minh.
"Regularized divergences between covariance operators and Gaussian measures on Hilbert spaces"
Journal of Theoretical Probability, volume 34, pages 580-643, 2021 - 9.
Ha Quang Minh.
"Infinite-dimensional Log-Determinant divergences between positive definite Hilbert-Schmidt operators".
Positivity (24), pages 631-662(2020) - 10.
Ha Quang Minh.
"A unified formulation for the Bures-Wasserstein and Log-Euclidean/Log-Hilbert-Schmidt distances between positive definite operators".
International Conference on Geometric Science of Information (GSI 2019), pages 475-483
Related Links
Lab Members
Principal investigator
- Minh Ha Quang
- Team Director
Core members
- Thanh Tam Le
- Visiting Scientist
Contact Information
Nihonbashi 1-chome Mitsui Building, 15th floor,
1-4-1 Nihonbashi, Chuo-ku, Tokyo
103-0027, Japan
Email: minh.haquang [at] riken.jp