RIKEN Center for Advanced Intelligence Project High-Dimensional Structure Theory Team
Team Director: Masaaki Imaizumi (Ph.D.)
Research Summary
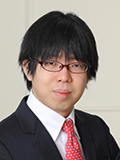
The High-Dimensional Structure Theory Team aims to deepen the understanding of data with high dimensionality and complex structures, together with the large-scale data science technologies needed to analyze them. To this end, we develop theoretical frameworks to describe high-dimensional settings. Leveraging tools from mathematical statistics, probability theory, and statistical mechanics, we tackle problems in modern data science, including high-dimensional statistics, deep learning theory, complex-data analysis, optimal transport, and causal inference.
Main Research Fields
- Informatics
Related Research Fields
- Mathematical & Physical Sciences
- Principles of Informatics/Statistical science
- Principles of Informatics/Mathematical informatics
Keywords
- High-dimensional statistics
- Deep learning theory
- Statistical learning theory
- Statistical causal inference
Selected Publications
- 1.
T.Tsuda, M.Imaizumi.:
"Benign Overfitting of Non-Sparse High-Dimensional Linear Regression with Correlated Noise"
Electronic Journal of Statistics, 18(2), (2024). - 2.
S.Nakakita, P.Alquier, M.Imaizumi.:
"Dimension-free Bounds for Sum of Dependent Matrices and Operators with Heavy-Tailed Distribution"
Electronic Journal of Statistics, 18(1), (2024). - 3.
J.Komiyama, M.Imaizumi.:
"High-dimensional Contextual Bandit Problem without Sparsity"
Advances in Neural Information Processing Systems, 36, (2023). - 4.
R.Zhang, M.Imaizumi, B.Schölkopf, K.Muandet.:
"Instrumental Variable Regression via Kernel Maximum Moment Loss"
Journal of Causal Inference, 11(1), (2023). - 5.
M.Imaizumi, J.Schmidt-Hieber.:
"On Generalization Bounds for Deep Networks Based on Loss Surface Implicit Regularization"
IEEE Transaction on Information Theory, 69(2), (2023). - 6.
M.Imaizumi, K.Fukumizu.:
"Advantage of Deep Neural Networks for Estimating Functions with Singularity on Hypersurface"
Journal of Machine Learning Research, 23(111), (2022), - 7.
R.Nakada, M.Imaizumi.:
"Adaptive Approximation and Generalization of Deep Neural Network with Intrinsic Dimensionality"
Journal of Machine Learning Research 21(174), (2020). - 8.
M.Imaizumi, K.Fukumizu.:
"Deep Neural Networks Learn Non-Smooth Functions Effectively"
PMLR: Artificial Intelligence and Statistics, (2019). - 9.
M.Imaizumi, T.Maehara, Y.Yoshida.:
"Statistically Efficient Estimation for Non-Smooth Probability Densities"
PMLR: Artificial Intelligence and Statistics, (2018). - 10.
M.Imaizumi, T.Maehara, K.Hayashi.:
"On Tensor Train Rank Minimization: Statistical Efficiency and Scalable Algorithm"
Advances in Neural Information and Processing Systems 30, (2018).
Related Links
Lab Members
Principal investigator
- Masaaki Imaizumi
- Team Director
Core members
- Noboru Isobe
- Special Postdoctoral Researcher
- Guillaume Braun
- Postdoctoral Researcher
- Hanna Tseran
- Postdoctoral Researcher
- Shogo Nakakita
- Visiting Scientist
- Hirofumi Ohta
- Visiting Scientist
- Ryo Okano
- Visiting Scientist
- Eshant English
- Student Trainee
- Le Milbeau Killian Antoine Morgan
- Student Trainee
- Mana Sakai
- Junior Research Associate
- Sota Nishiyama
- Research Part-time Worker II
Contact Information
Nihonbashi 1-chome Mitsui Building, 15th floor,
1-4-1 Nihonbashi, Chuo-ku, Tokyo
103-0027, Japan
Email: masaaki.imaizumi@riken.jp